From Machine Learning to Nowcasting
From Machine Learning to Nowcasting
WONG Wai-kin
September 2020
Artificial intelligence (AI), machine learning and deep learning have been evolving rapidly in various fields during the recent years. For heavy rain forecasts, is it possible for AI to replace weather forecasters?
Weather changes are governed by the laws of physics. Meteorological agencies around the world use a variety of instruments to monitor weather elements – such as wind, temperature, humidity and pressure measured directly from surface weather stations, radiosondes and aircraft; rainfall intensity and locations from Doppler weather radar; and cloud images from multi-spectral imagers on-board of meteorological satellites. Forecasters make use of massive real-time weather data to analyse and monitor current weather condition and its trend. Meanwhile, the observational data are input to the numerical weather prediction models running on computers to project weather changes. The computer model outputs are provided to forecasters for reference or utilized directly to generate automatic forecast products. However, the accuracy of the computer model forecast is limited by many factors. In particular, there remains considerable room for improvement in forecast of fast-changing and small-scale weather phenomena such as heavy rain and thunderstorms[1].
The meteorological communities have been applying AI for decades in support of analysis and forecast of weather. In SWIRLS nowcasting system developed and operated by the Observatory, the core component is indeed based on object tracking method from computer vision technique of AI. Radar echoes are forecast by extrapolating rain areas and thunderstorms for the next few hours. However, the intensity of the rain area and motion field are assumed unchanged in this method[2].
In reality, development of rainstorm can be extremely rapid, where movement and intensity change of the thunderstorms and rain areas vary significantly within a few minutes or so. If the characteristics of the variations in thunderstorms and rain areas can be obtained from radar images during the past heavy rain situations, they will be useful to improve forecasting the growth and decay of rainstorm. The deep learning method makes use of radar image data over past many years in training to analyse the characteristics of the rainfall in space and time. The deep learning method also looks for optimized algorithms to establish an effective neural network to register features about the change of radar rainfall intensity and movement during the past weather processes. When new radar image is available, the deep learning model will identify rain areas from this current image and compare their changes over the recent radarscopes. The features stored in neural network are then applied to generate rainfall distribution and intensity forecast for the next couple of hours[3] ( Figure 1).
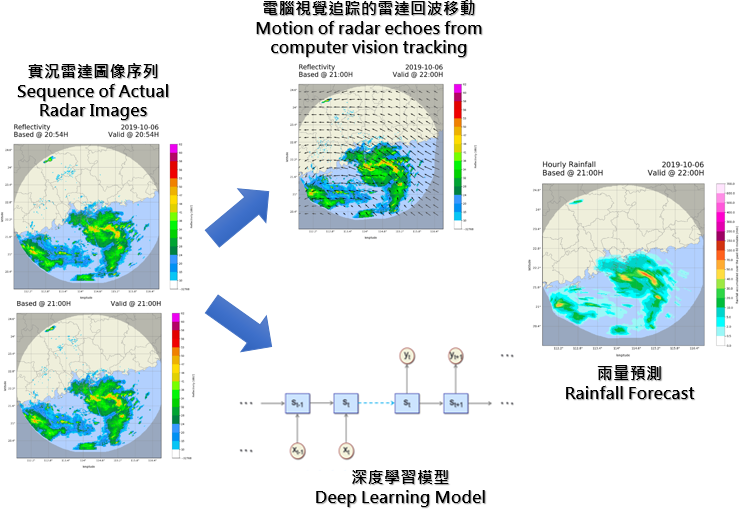
Figure 1. Rainfall nowcasting using AI computer vision versus deep learning approach.
In recent years, the Observatory has been cooperating with universities to develop deep learning technique to enhance rainfall nowcasting. From the results of real-time trial, the deep learning model has considerable impact on improving the performance of heavy rain forecast in the next one to two hours. At present, the accuracy of machine learning in forecasting rainstorms or other weather phenomena relies on appropriate computation models to identify the characteristics or features of weather changes. The training process requires a large amount of past data. More abundant and diversified training datasets can improve the capability of neural network in identifying different weather scenarios from the data. The latest research on deep learning models has also actively used or incorporated physical laws governing weather changes to improve heavy rain forecasts, or to explore applications in forecasting extreme weather. The reliability and stability in performance of deep learning forecast products remain to be verified. Moreover, AI, machine learning and deep learning can undoubtedly provide more valuable reference and forecasting tools derived from meteorological big data that can support forecasters to enhance or develop meteorological forecasts and warning services.